Understanding Scatterplots in ABA
Visual Representation of Data
In the field of Applied Behavior Analysis (ABA), a scatterplot serves as a vital tool for visually representing data. It displays the relationship between two variables, which helps professionals analyze behavioral assessments or interventions [1]. A scatterplot allows practitioners to plot data points on a graph, where one variable is represented on the x-axis and another on the y-axis. This graphical method can help simplify complex data, making it easier to understand and interpret.
Scatterplots can reveal trends, patterns, and correlations within behavioral data. The visual nature of scatterplots aids in quickly identifying distributions and outliers within the data set. Understanding these visual representations is critical for developing effective intervention strategies.
Variable X (e.g., Frequency of Behavior)Variable Y (e.g., Time of Day)1225374356
Purpose of Scatterplots
The primary purpose of scatterplots in ABA is to aid professionals in identifying patterns and trends within the data collected. By visually examining these relationships, practitioners can assess various behavioral dynamics such as increases or decreases in problematic behaviors, or the effectiveness of certain interventions.
Scatterplots also highlight different types of relationships, including positive correlation, negative correlation, or no correlation. This analysis is crucial for understanding behavior and enhancing intervention strategies. For instance, an upward trend may indicate improvement, while a downward trend suggests a decline [3].
Using scatterplots, ABA professionals can track progress over time, evaluate the impact of interventions, and make informed decisions based on the data displayed. As practitioners become adept at interpreting scatterplot data, they gain the ability to implement more effective and individualized treatment plans for clients.
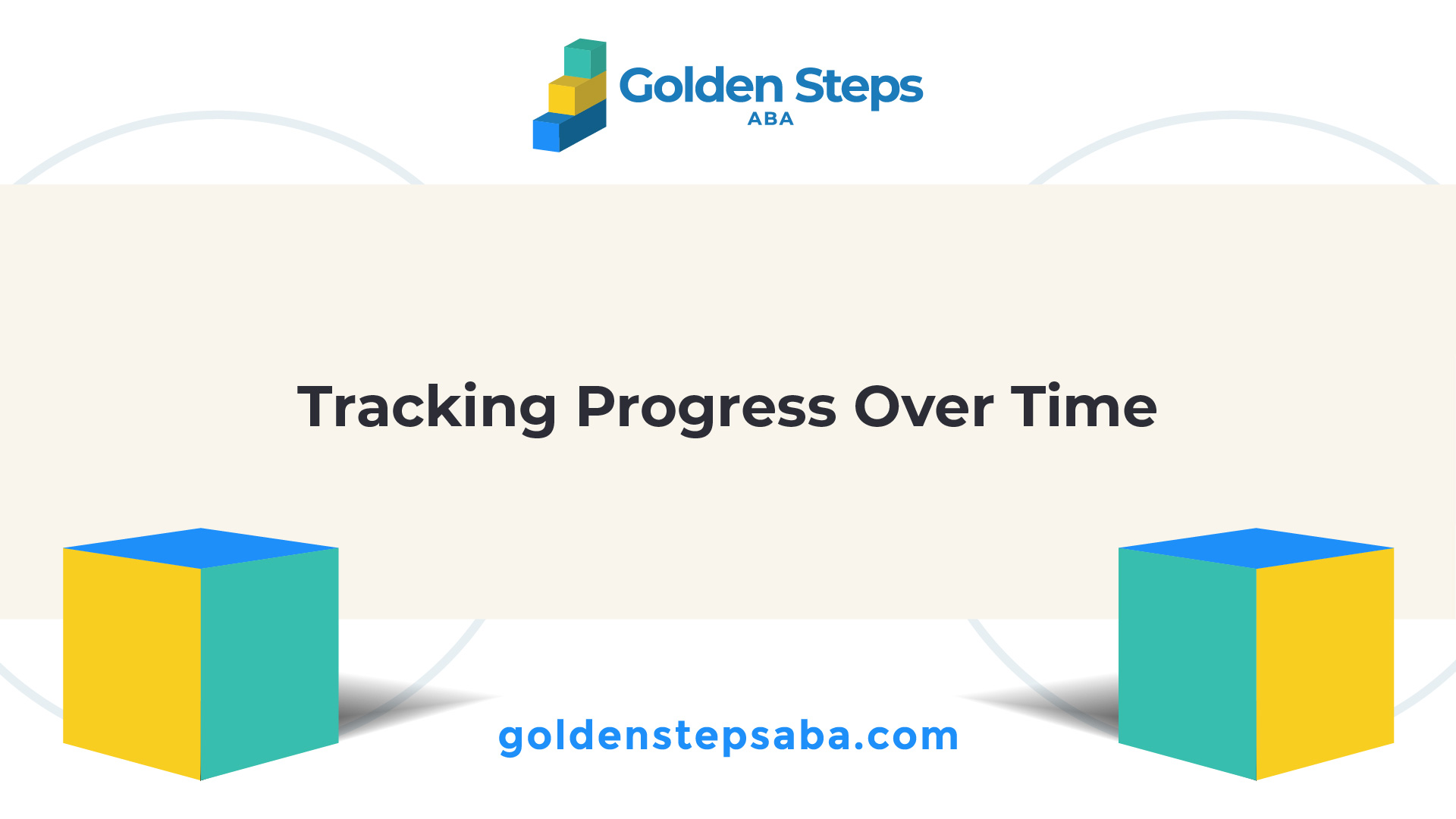
Analyzing Data with Scatterplots
Identifying Trends and Patterns
In Applied Behavior Analysis (ABA), scatterplots serve as a critical tool for identifying trends and patterns within behavior data. Understanding scatterplots can help professionals observe whether behaviors are improving or declining over time. An upward trend in a scatterplot indicates an improvement in behavior, while a downward trend signifies a decline. This visual representation allows behavior analysts to discern not just the overall progress but also specific fluctuations that may demand attention [3].
Outliers are another important aspect of scatterplots. They can provide significant insights into factors influencing behavior. For instance, if a data point is markedly different from others, it may indicate the impact of a specific variable or trigger, allowing for a more effective intervention strategy. By understanding such trends, professionals can better tailor their behavior intervention plans to meet the needs of individuals.
Assessing Relationships between Variables
Scatterplots excel at visually representing the correlation between two variables. In ABA, this can help practitioners identify patterns that may not be evident through traditional data collection methods. There are three main types of relationships illustrated:
By analyzing these relationships through scatterplots, professionals can gain a deeper understanding of behaviors, factors that influence them, and ultimately make more informed, data-driven decisions regarding interventions. For a more comprehensive approach, combining scatterplot analysis with other forms of data collection is recommended.
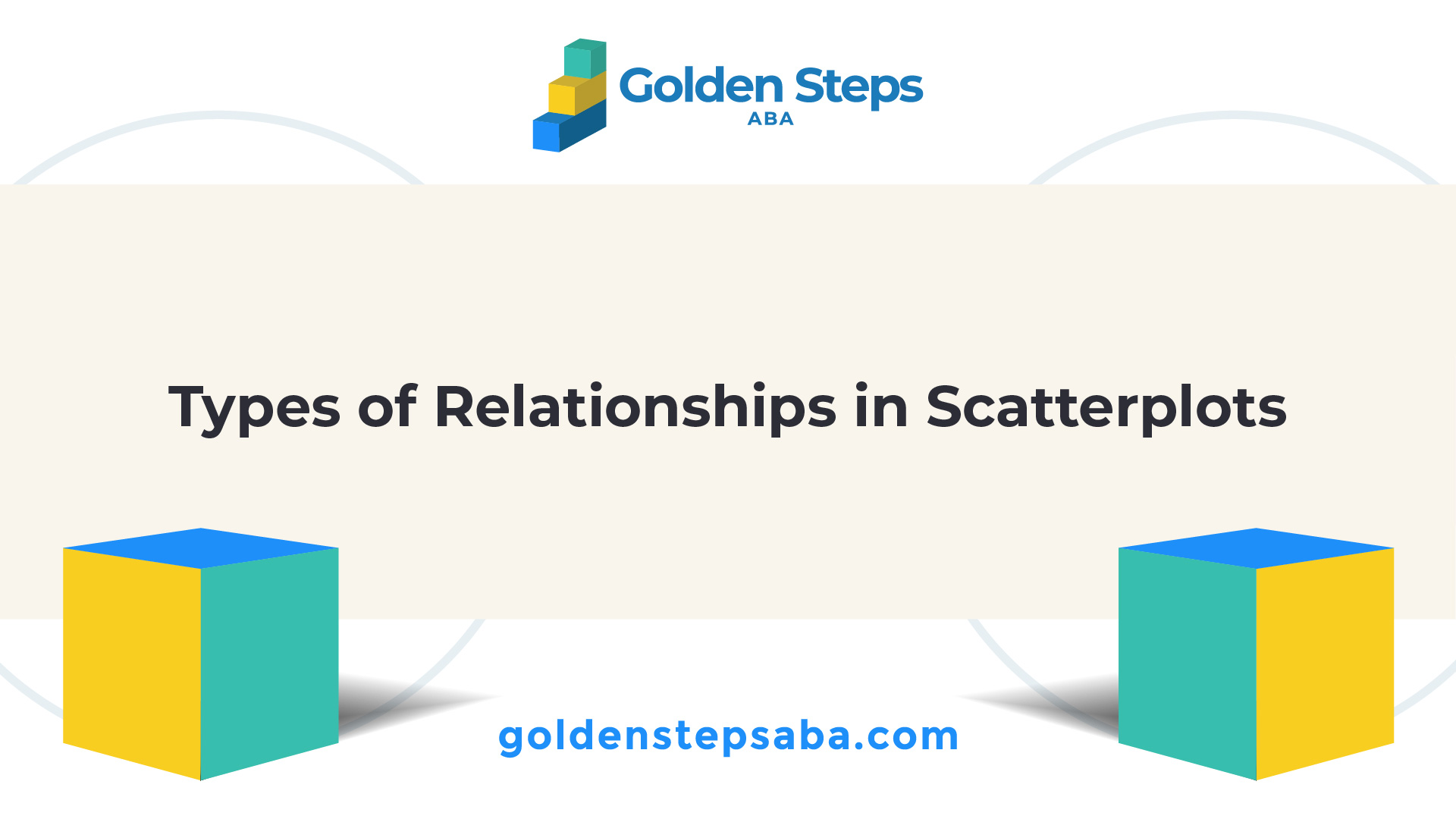
Types of Relationships in Scatterplots
Scatterplots are valuable tools in Applied Behavior Analysis (ABA) for visualizing relationships between variables. Understanding these relationships helps in identifying trends that can inform intervention strategies.
Positive Correlation
In a scatterplot, a positive correlation indicates that as one variable increases, the other variable also tends to increase. This suggests a direct relationship between the two associated variables. For example, if the number of positive reinforcements given to a child increases, their compliance with requests may also rise.
Variable A (Reinforcements)Variable B (Compliance)13253749
This table illustrates that with an increase in reinforcements, compliance also improves. Visualizing this relationship in a scatterplot highlights the upward trend.
Negative Correlation
Conversely, a negative correlation occurs when one variable increases while the other variable decreases. This indicates an inverse relationship between the two variables, suggesting that as one factor goes up, the other tends to decline. For example, consider a scenario where increased distractions in a learning environment correlate with decreased attention from a student [4].
Variable A (Distractions)Variable B (Attention Level)18263442
The table above shows that as distractions increase, attention levels drop, illustrating a downward trend on a scatterplot.
No Correlation
No correlation implies that there is no apparent relationship between the variables. In this case, the data points are scattered randomly across the plot, with no discernible trend. For instance, the hours spent on leisure activities do not affect the ability to follow instructions in a structured setting.
Variable A (Leisure Hours)Variable B (Instruction Following)15243643
The data in the table indicate that changes in leisure hours do not correlate with the ability to follow instructions. This randomness is depicted through scattered data points without any definitive upward or downward trend in the scatterplot.
By analyzing these different types of correlations within scatterplots, professionals in ABA can gain insights into relationships between variables. For a deeper understanding of how scatterplots enhance data interpretation in therapy, refer to operation definitions in ABA.
Creating Effective Scatterplots
Creating effective scatterplots is a fundamental skill in Applied Behavior Analysis (ABA). This involves a systematic approach to data collection and a clear understanding of how to accurately plot and label variables.
Data Collection Process
The data collection process is a critical first step when preparing to create a scatterplot. Data should be gathered systematically to ensure accuracy and reliability. Typically, this involves observing behavior and recording relevant variables that need to be analyzed.
A simple table can illustrate typical data collection for a scatterplot:
Session NumberIndependent Variable (e.g., Intervention Time)Dependent Variable (e.g., Number of Target Behaviors)115 mins3230 mins5325 mins4420 mins6535 mins7
When collecting data, it is essential to define the operational definitions of both the independent and dependent variables involved. This ensures that everyone involved in the data collection process understands what is being measured. Once sufficient data is collected, professionals can begin to prepare their scatterplot.
Plotting and Labeling Variables
The next step in creating a scatterplot is plotting and labeling the variables accurately. The independent variable is typically represented on the x-axis, while the dependent variable is plotted on the y-axis. Following the initial preparation of data, these variables are plotted on a graph.
When creating a scatterplot, professionals often leave data points as individual marks rather than connecting them with lines, unless a trendline is being considered. Adding a trendline can help visualize any linear patterns or associations between the two variables.
Here are some key tips for plotting and labeling:
Using scatterplots effectively allows practitioners in ABA to identify different types of relationships between variables, critical for developing effective intervention strategies. Correctly plotted scatterplots provide visual evidence of changes in behavior over time, making them a valuable tool in the therapeutic process. For more information about behavior analysis and its practical applications, see articles on operational definitions in ABA or positive reinforcement in ABA therapy.
Utilizing Scatterplots in ABA
Scatterplots serve as a crucial tool in Applied Behavior Analysis (ABA), allowing professionals to track progress and make adjustments to intervention strategies based on visual data representation.
Tracking Progress Over Time
ABA scatterplot analysis facilitates monitoring progress by graphing data points to visualize the change in behavior over time. This method enables practitioners to observe trends that indicate improvement or decline. For example, an upward trend in a scatterplot suggests that the target behavior frequency is increasing, while a downward trend indicates a reduction in that behavior.
To illustrate tracking progress, consider a hypothetical case study where a child is learning to communicate using verbal expressions. Below is a simplified representation of data collected over a 10-week period:
WeekFrequency of Verbal Expressions1325374859687108129131015
Using this data, a scatterplot can be created where the x-axis represents the weeks and the y-axis shows the frequency of verbal expressions, allowing for an easy visual reference of progress over time. By understanding how behaviors evolve during the intervention, practitioners can affirm the effectiveness of their strategies and remain agile in their approach.
Modifying Intervention Strategies
In addition to tracking progress, scatterplots allow for the identification of outliers and trends that can inform modifications to intervention strategies. Outliers in a scatterplot represent data points that deviate significantly from the general trend, providing insight into behaviors that may be influenced by specific triggers or external factors.
By analyzing these outliers, professionals can adjust intervention strategies to better meet the needs of the individual. For instance, if an unexpected drop in the frequency of a desired behavior occurs at a specific session, further investigation may reveal changes in the environment or modifications in routine that could have impacted the outcome.
The use of scatterplots enables practitioners to make data-informed decisions about treatment plans. By continuing to analyze behavioral patterns and the effectiveness of various interventions, they can hone in on optimal approaches for various situations. This approach not only enhances the quality of care for individuals but also empowers parents and caregivers with a clear understanding of progress and strategies in place.
For more information on effective data analysis techniques in ABA, consider exploring topics such as positive reinforcement in ABA therapy, what is precision teaching in ABA therapy?, and operational definitions in ABA.
Importance of Scatterplot Analysis
Empowering Parents and Professionals
Scatterplot analysis in ABA (Applied Behavior Analysis) plays a pivotal role in empowering both parents and professionals involved in therapy. By utilizing visual representations of behavioral data, stakeholders can actively participate in the intervention process. This analysis helps determine if interventions are yielding effective results, tracking progress over time, and identifying necessary modifications in intervention plans [3].
Visual insights into behavior patterns allow parents to become more informed advocates for their children. They can recognize trends and patterns, enabling them to engage meaningfully in discussions about treatment strategies. This collaborative approach fosters a partnership between caregivers and practitioners, leading to improved outcomes for individuals with autism and other developmental challenges.
Making Data-Informed Decisions
Data-informed decision-making is crucial in ABA therapy. Scatterplots facilitate this by providing clear visualizations of behavioral progress which highlight potential challenges over time. These insights enable professionals to adjust intervention strategies as needed, ensuring that they remain effective and appropriately tailored to individual needs.
By interpreting scatterplots, parents and professionals can set realistic goals based on patterns identified in the data. They can celebrate progress, which reinforces positive reinforcement strategies. Additionally, understanding whether relationships between variables show positive, negative, or no correlation further enriches the decision-making process. For further information on behavioral strategies, exploring topics such as what is the Premack principle? and what is precision teaching in aba therapy? may be beneficial.
The ability to analyze data effectively not only enhances the treatment process but also empowers everyone involved to achieve meaningful outcomes. By engaging with scatterplot analysis, parents and professionals alike are well-equipped to navigate the complex landscape of intervention in ABA therapy.